How AI is remodelling the IoT
Monday, 17 March, 2025 | Supplied by: Nordic Semiconductor
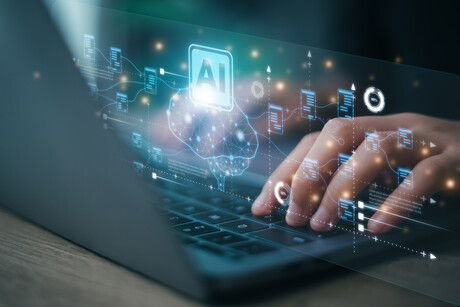
Back in 1956, Allen Newell, Cliff Shaw and Herbert Simon put together a computer program called The Logic Theorist. Funded by the RAND Corporation, the program intended to mimic the problem-solving skills of a human and is considered by many to be the first example of artificial intelligence (AI).
Fast forward to today, and the poster child for AI is the large language model (LLM). LLMs are computational algorithms that achieve general-purpose language generation and other natural language processing tasks by learning statistical relationships from text documents during an intensive training process. OpenAI’s ChatGPT is perhaps the best-known example.
Useful though they are, for the IoT it is not the potential of LLMs that’s driving things forward. Where things get exciting is when huge volumes of data collected by automated systems are used to train machine learning (ML) algorithms that are later deployed to make IoT devices make better and smarter decisions. ML is an application of AI that involves training algorithms on large datasets, enabling computers to make predictions or decisions based on patterns and insights derived from the data, without being explicitly programmed to perform specific tasks. This process enhances the capabilities and intelligence of machines over time as they process more data.
The IoT can be described as a global interconnected network with the capacity to collect a virtually limitless volume of data. That data can be fed to computers in the cloud and used to fuel ML algorithms that enhance the intelligence of the billions of connected devices making up the network. Even the most modest IoT device can become continually smarter, offering huge potential for the future of industry, commerce, education, medicine and more.
ML in action
Despite a range of tough engineering challenges, developers are already introducing IoT products with ML into the commercial sector. One example is Norwegian company Sensorita. The firm has launched a smart waste management solution based on Nordic’s nRF9160 SiP cellular IoT product. Sensorita’s device uses radar technology based on research from the Norwegian University of Life Sciences to assess the fill levels and contents of large waste receptacles.
Waste management companies face problems such as customers moving waste disposal containers and disposing of different waste than agreed, not knowing how full the container is, what’s in it or when it should be picked up. This creates problems for logistics and production planning, and increased CO2 emissions from unnecessary pick-ups.
Sensorita has addressed the problem with a rugged sensor incorporating radar and GPS. The sensor takes radar images of the inside of the container multiple times per hour, and these are then sent to the Sensorita cloud platform for analysis. Using ML algorithms trained on millions of radar images, the sensor can estimate how full the receptacle is, and the main material type it contains.
The nRF9160 SiP uses cellular network location data and GNSS trilateration to record the precise location of each container and transmits the sensor data to the Sensorita cloud platform through its LTE-M/NB-IoT modem. Sensorita also uses ML to optimise the route taken by trucks as they travel around cities emptying the waste containers; the results are fuel savings, reduced man-hours and lower carbon emissions.
More efficient fridges, predictive maintenance and waste bin optimisation are important, but they are niche. When ML is widely deployed on the network the results will be dramatic. One example is the impact of the technology on health care. Wireless SoCs like Nordic’s nRF54H20 will support wearables with a wide array of sensors such as heart rate, heart rate variability (HRV), temperature, respiration rate, blood oxygen, stress, fatigue and other physiological signs. Such wearables won’t be just for fitness fanatics, but worn by seniors, adults and children alike.
Armed with ML and cellular IoT connectivity, the wearable becomes a device that can continuously monitor multiple vital signs simultaneously. And if some or all show signs of change, the wearable’s ML model could determine if the trend is trivial or a serious medical emergency. An example might be a sudden change in blood oxygen level, heart rate, blood pressure and respiration rate that indicate an imminent cardiac problem. If things are serious, the wearable could notify first responders while also providing them with vital data ahead of arrival.
Such a device could protect stretched healthcare budgets by cutting down on hospital visits while optimising the care of people who really need it. That would save hundreds of billions of dollars across the world.
The ability to perform ML on low-power, embedded devices that can run on batteries will transform the IoT. It will make the network even more intelligent, capable and flexible. And it will enable new types of products and applications that were previously impossible. It makes for an exciting future and one which is much closer than you might think.
Phone:
Yamaha YsUP software for SMT machinery
The YsUP software for SMT machinery enables PCH manufacturers to overcome the challenges of...
Siemens Process Preparation Stencil Engineering Tool
The Siemens Process Preparation Stencil Engineering Tool is designed to provide first-time-right...
Nordic Semiconductor nPM2100 Power Management IC
The nPM2100 PMIC features an efficient boost regulator and a range of energy-saving features to...